
Limitations of the algorithms
Any algorithm is at most a simplified model of real-world phenomena. Therefore, it can never fully capture all possible factors that are critical to understand disease states. To this end, the outcomes of our algorithms shall be treated with care and cannot be considered by any means a perfect reflection of real clinical pictures.

Any algorithm is at most a simplified model of real-world phenomena. Therefore, it can never fully capture all possible factors that are critical to understand disease states. To this end, the outcomes of our algorithms shall be treated with care and cannot be considered by any means a perfect reflection of real clinical pictures.
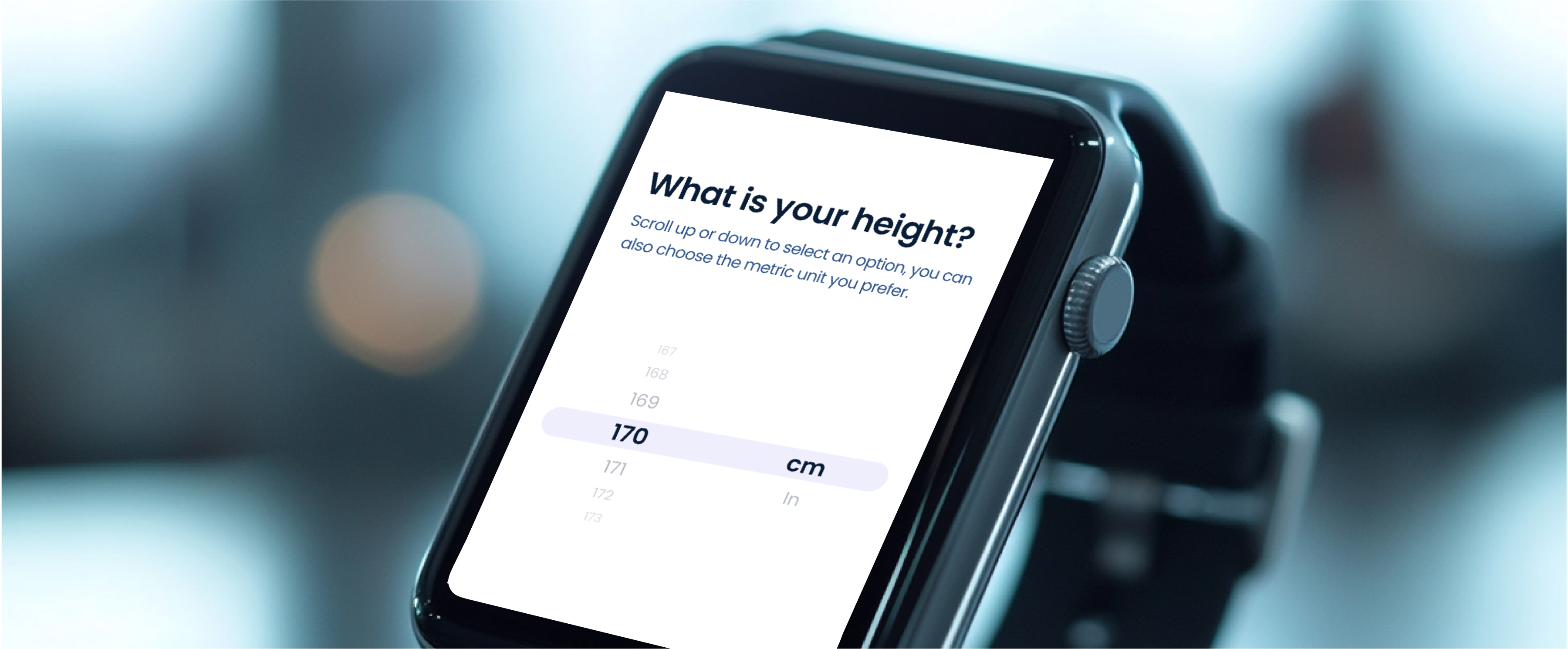
In general, the quality and accuracy of the output of an SaMD depends directly on the quality of input data. For our algorithms, two factors are primarily affecting the quality of calculated scores. Firstly, the data collected from smartwatches is not as accurate and precise as properly calibrated medical devices in clinical environments. Thus, the input data generally is less reliable and cannot replace clinical investigations. Secondly, if the smartwatches are not worn or worn properly, or issues with sensors, batteries etc of the watches are present, the algorithm will be exposed to missing data that will affect its efficacy.
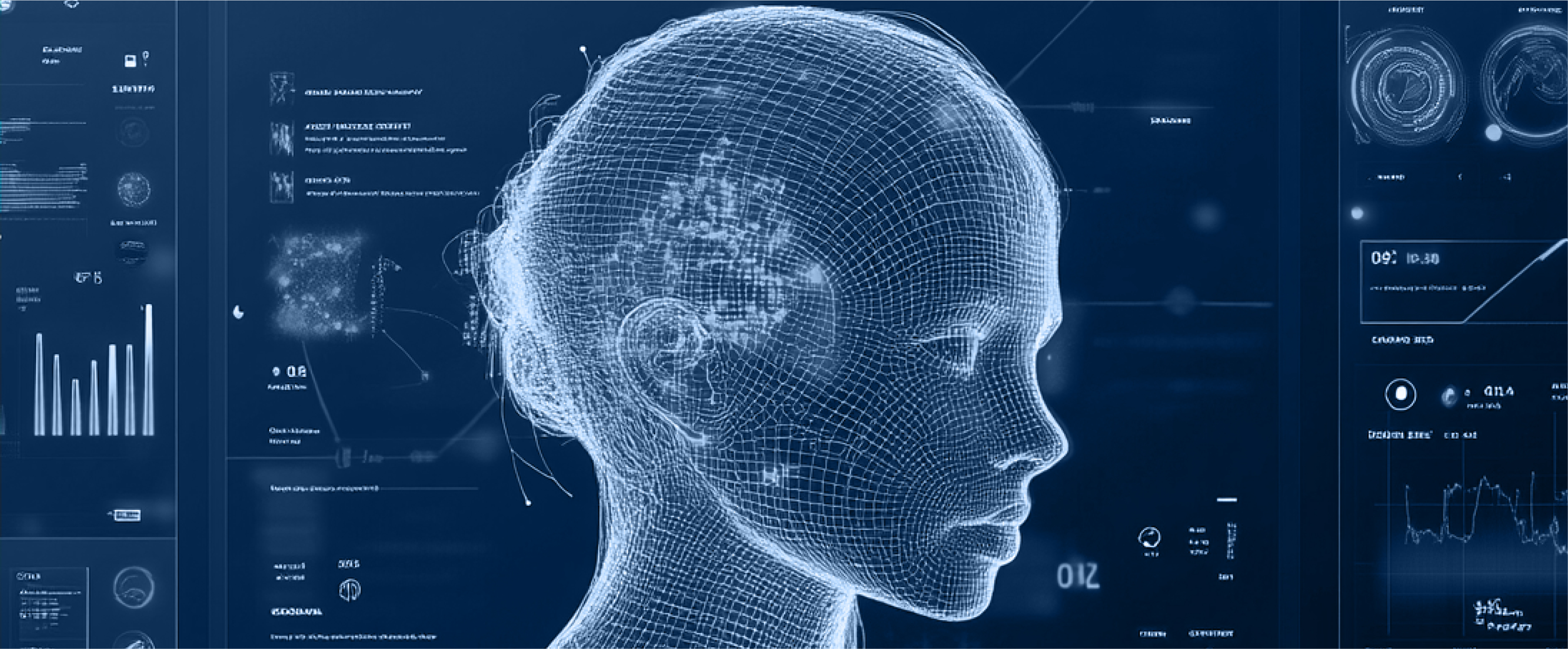
The past decades have been witnessing a major trend towards evidence-based classification of psychiatric disorders using measurable dimensions. The EERLYY app was the result of a major effort to use continuous longitudinal measurements of biobehavioral variables to characterize substance use disorders in a quantitative manner and relapse as a transition between states.